New Machine Learning Strategy for Optimizing Interventions in Causal Model Design
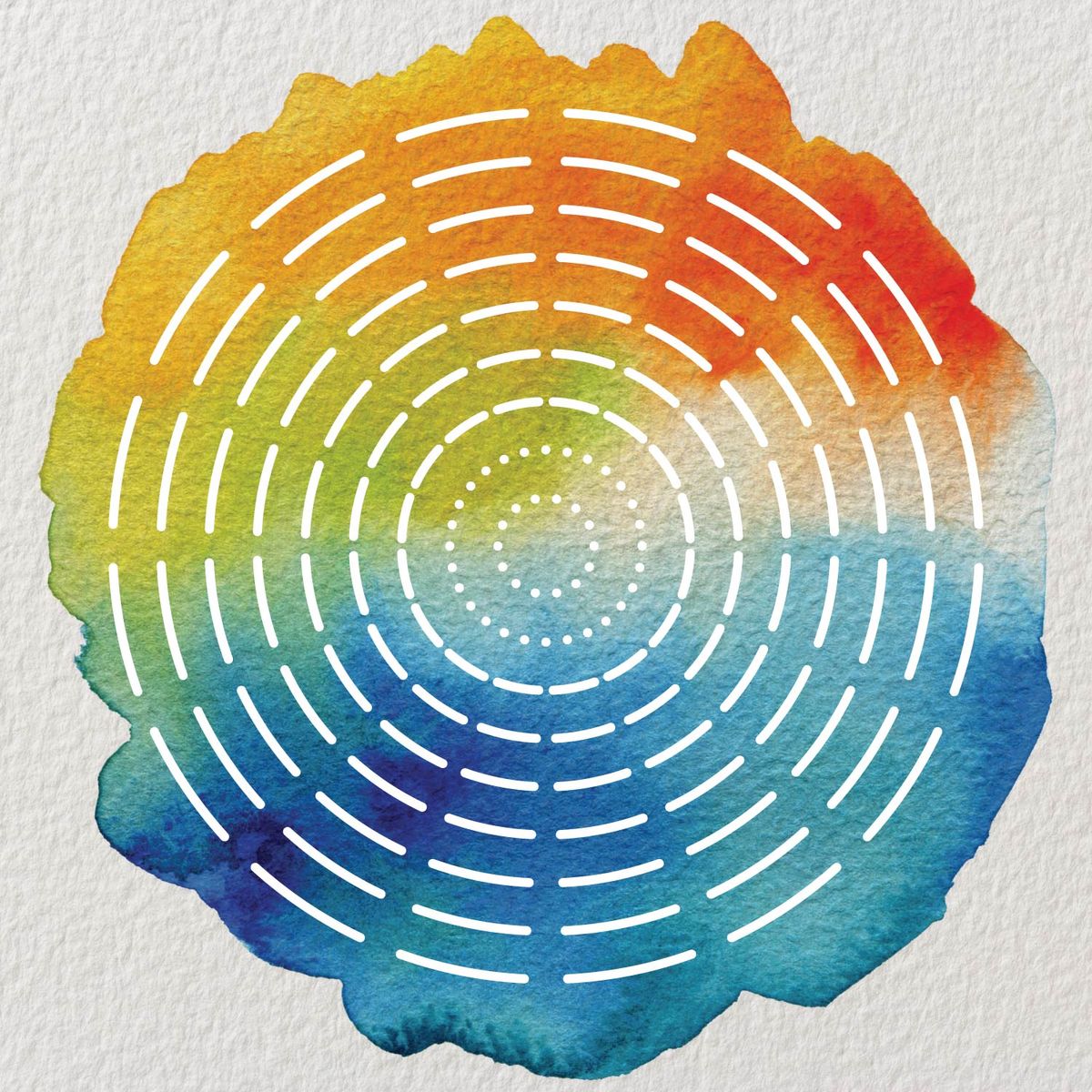
Researchers developed a new active learning—or machine learning—strategy that outperformed existing approaches for identifying optimal interventions when designing causal models. The new approach, which was developed by researchers from Massachusetts Institute of Technology and Harvard University, was recently described in a paper in Nature Machine Intelligence. The research was partially funded by the National Center for Complementary and Integrative Health.
Identifying interventions that can be applied to a system to produce a desired outcome is a challenge across many disciplines, including science, engineering, and public policy. Not knowing much about an outcome before implementing an intervention can mean extensive options for the intervention design, and doing an exhaustive search for the optimal design may not be feasible. In such cases, when the number of interventions is large, experimental design strategies are needed to help identify desirable interventions more efficiently.
Standard correlation-based approaches do not represent the causal relationships between the interventions and the outcomes. Causal-based models are needed to understand the impact of interventions on the desired outcomes and identify the desirable interventions more efficiently. In the development of this new machine learning strategy, researchers applied an updated Bayesian statistical approach to the causal model and prioritized interventions through a causally informed intervention acquisition function that allowed for fast optimization.
First, researchers modelled and updated the edge weights in the causal model by using the Bayesian approach of directed acyclic graph (DAG)-Bayesian linear regression (BLR) distribution. Then, they used a class of causally aware acquisition functions to select the next best intervention. The underlying causal structure was respected by the DAG–BLR distribution, allowing for efficient posterior updates (i.e., adding samples from the next best intervention), while tractable closed-form evaluations within the acquisition function resulted in efficiently optimizing the selection of the next intervention to add to the model.
The researchers applied the new strategy to both synthetic data and a single-cell gene expression dataset. The new approach outperformed existing empirical analogues in both cases, resulting in accurate predictions that required fewer experiments.
Although the researchers related their work to the area of cellular reprogramming, they think the new causal active learning strategy can be applied broadly to sequential design problems that occur in complex systems, including in fluid mechanics, dynamic pricing, and cancer immunotherapy.
Reference
- Zhang J, Cammarata L, Squires C, et al. Active learning for optimal intervention design in causal models. Nature Medicine Intelligence. October 2, 2023. [Epub ahead of print].
Additional Resources
NIH Strategically, and Ethically, Building a Bridge to AI (Bridge2AI)
Publication Date: October 2, 2023